Noam Auslander, Ph.D.
-
Assistant Professor, Molecular and Cellular Oncogenesis Program, Ellen and Ronald Caplan Cancer Center
Auslander focuses on developing machine learning methods to understand genetic and infectious factors that drive cancer development and identify patterns that can improve cancer diagnosis and treatment.
Auslander earned her B.S. in computer science and biology from Tel Aviv University and continued her studies in Maryland, where she obtained a computer science Ph.D. from the University of Maryland with a combined fellowship at the National Cancer Institute. She received postdoctoral training at the National Center of Biotechnology Information (NCBI) and joined The Wistar Institute in 2021 as an assistant professor.
The Auslander Lab
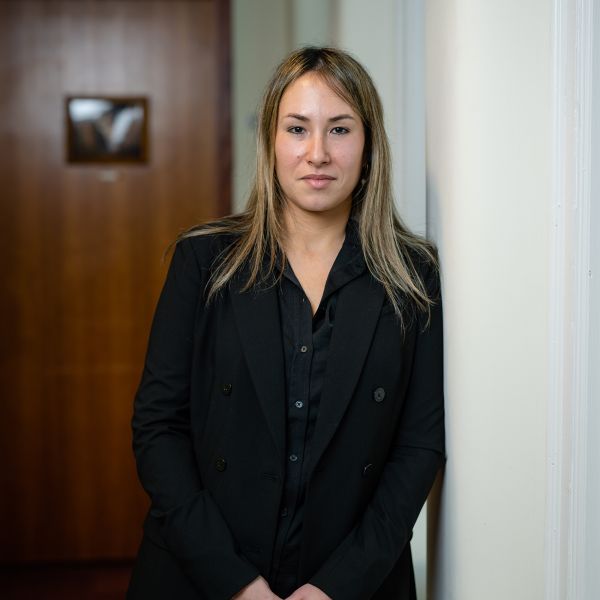
The Auslander Lab
In the past years, enormous growth in the quantity of biomedical data has been paralleled by advances in computational and deep learning techniques. These rapidly accumulating biomedical and clinical datasets have the potential to uncover unknown genetic drivers of cancer, carcinogenic infectious agents, and new treatment biomarkers. To achieve this potential, there is a need for improved and more interpretable computational strategies that can handle different types of biomedical data.
The Auslander laboratory develops advanced machine and deep learning methods to identify factors that drive cancer development and predict patients’ prognoses. We focus on the development of deep learning methods to identify new infectious agents in cancer, and the development of machine learning strategies that improve outcome prediction through biologically interpretable classifiers.
-
Postdoctoral Fellow
Abdurrahman Elbasir, Ph.D.
-
Graduate Students
Anastasia Lucas
Andrew Patterson
Julia C Malnak (Rotation student, UPenn-GCB) -
Bioinformatics Technician
Bryant Duong
-
Research Assistants
McKenna Reale
Bryant Duong
-
Postdoctoral Fellow
Abdurrahman Elbasir, Ph.D.
-
Graduate Students
Anastasia Lucas
Andrew Patterson
Julia C Malnak (Rotation student, UPenn-GCB) -
Research Assistants
McKenna Reale
Bryant Duong
Research
Characterization of the Tumor Microbiome with Machine Learning
Detection of new tumor microbes can advance early diagnosis and cancer prevention strategies. Our lab develops machine learning and sequence analysis frameworks to characterize the landscape of microbes that underlie different cancer types and modulate patient outcomes in cancer.
Quantification of Gut Microbial Genes and Development of Fecal Biomarkers
The gut microbiome has immense potential for improving cancer clinical decision-making through non-invasive fecal biomarkers. Our lab develops methods that improve quantification of microbial genes to allow reliable fecal biomarkers for cancer.
New Virus Characterization and Detection of Pathogenic or Immunogenic Viral Sequences
The human virome is highly heterogenous with numerous uncharacterized members. Our lab develops integrative machine learning and sequences analysis methods to identify new viruses, viral genes, or genomic regions that are associated with disease risk, severity, and immune responses.
Biologically Informed Classifiers of Cancer Treatment Responses
A major difficulty limiting the translational potential of machine learning methods is low biological interpretability. Our lab develops biologically motivated classifiers of cancer outcomes by incorporating biological knowledge, networks, and databases with cancer omics.
Auslander Lab in the News
Selected Publications
A Deep Learning Approach Reveals Unexplored Landscape of Viral Expression in Cancer.
Elbasir, A., Ye, Y., Schäffer, D.E., Hao, X., Wickramasinghe, J., Tsingas, K., Lieberman, P.M., Long, Q., Morris, Q., Zhang, R., et al. “A Deep Learning Approach Reveals Unexplored Landscape of Viral Expression in Cancer.” Nat Commun. 2023 Feb 11;14(1):785. doi: 10.1038/s41467-023-36336-z.
Mutated Processes Predict Immune Checkpoint Inhibitor Therapy Benefit in Metastatic Melanoma.
Patterson A., Auslander N. “Mutated Processes Predict Immune Checkpoint Inhibitor Therapy Benefit in Metastatic Melanoma.” Nat Commun. 2022 Sep 19;13(1):5151. doi: 10.1038/s41467-022-32838-4.
In silico learning of tumor evolution through mutational time series.
Auslander, N., Wolf, Y.I., Koonin, E.V. “In silico learning of tumor evolution through mutational time series.” Proc Natl Acad Sci U S A. 2019 May 7;116(19):9501-9510. doi: 10.1073/pnas.1901695116.
Seeker: alignment-free identification of bacteriophage genomes by deep learning.
Auslander, N., Gussow, A.B., Benler, S., Wolf, Y.I., Koonin, E.V. “Seeker: alignment-free identification of bacteriophage genomes by deep learning.” Nucleic Acids Res. 2020 Dec 2;48(21):e121. doi: 10.1093/nar/gkaa856.
Robust prediction of response to immune checkpoint blockade therapy in metastatic melanoma.
Auslander, N., Zhang, G., Lee, J.S., Frederick, D.T., Miao, B., Moll, T., Tian, T., Wei, Z., Madan, S., Sullivan, R.J., et al. “Robust prediction of response to immune checkpoint blockade therapy in metastatic melanoma.” Nat Med. 2018 Oct;24(10):1545-1549. doi: 10.1038/s41591-018-0157-9. Epub 2018 Aug 20.